Has the king been dethroned?
Since March 2023, GPT-4 has stood as the undisputed leader among Large Language Models, a significant leap ahead of its predecessors and a benchmark for new entrants. Competitors have often been judged successful if they managed to surpass GPT-3.5, underlining the advanced nature of GPT-4. Yet, the recent announcement of Google's Gemini model could signal a change in this dynamic. Gemini's groundbreaking approach to multimodal processing, integrating image, audio, video, and text data, sets it apart in the field of AI. Reports suggest that Gemini outperforms GPT-4 in several benchmarks, yet its introduction has been mired in controversy. Criticisms have emerged over Google’s presentation, which included a video that overstated the model's capabilities and their blog post, which downplayed instances where GPT-4 still held the upper hand.
Training Data and Architecture
Gemini models are built upon Transformer decoders, but with enhancements in architecture and model optimization. These improvements are crucial for enabling stable training at large scales and for optimized performance on Google’s Tensor Processing Units (TPUs), enabling the handling of a 32k token context length.
A key aspect of Gemini's design is its multimodal training regimen, which incorporates a diverse blend of data including images, audio, video, and text. This approach allows Gemini to engage with a broader spectrum of information types, providing it with a more versatile toolkit compared to traditional text-centric LLMs. By integrating these varied data formats, Gemini offers a more rounded and adaptable AI model, advancing the field of Large Language Models with its practical and inclusive data handling capabilities.
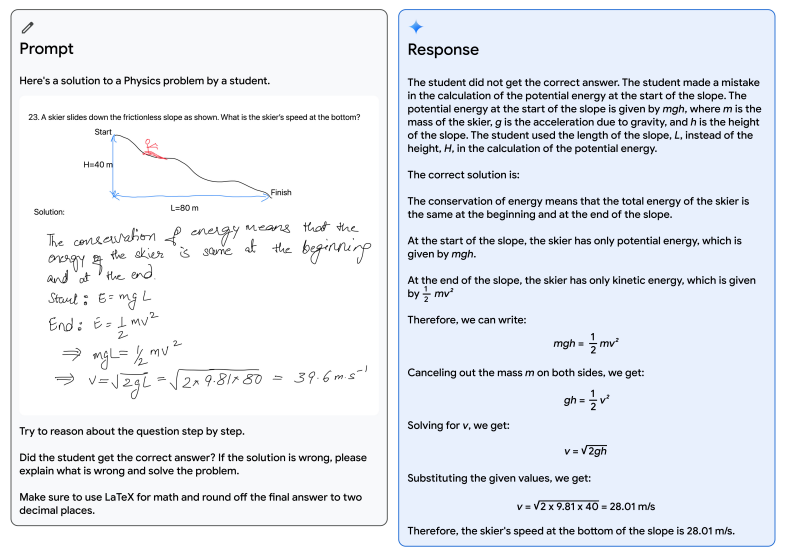
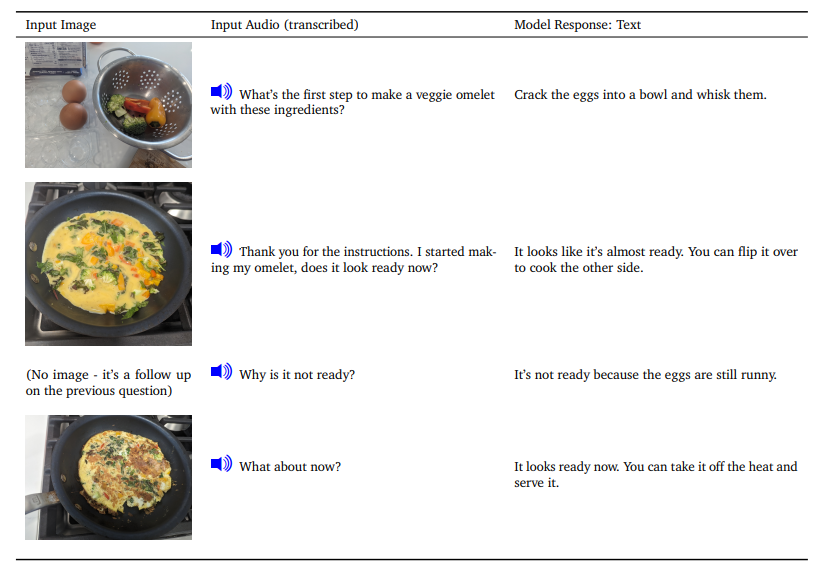
Different Versions of the Model
The Gemini model family is designed to cater to a wide array of applications and computational needs, from complex reasoning to on-device applications, manifesting in three distinct versions: Ultra, Pro, and Nano. Each of these versions is uniquely tailored to meet specific performance and deployment criteria:
- Gemini Ultra: Represents the pinnacle of the Gemini series in terms of capabilities. Gemini Ultra is engineered for handling highly complex tasks, setting new benchmarks in performance across a diverse range of applications, including advanced reasoning and multimodal tasks. Its architecture is optimized to deliver state-of-the-art performance while being efficiently serveable at scale on TPU accelerators. The Ultra model's proficiency in a wide spectrum of demanding benchmarks underlines its position as the most capable model in the Gemini family.
- Gemini Pro: Positioned as a performance-optimized model, Gemini Pro strikes a balance between cost, latency, and high performance. It exhibits strong reasoning performance and broad multimodal capabilities, making it a versatile choice for scalable deployment. The Pro model is designed to be a more accessible yet powerful option within the Gemini family, providing significant performance across a range of tasks while being mindful of resource utilization.
- Gemini Nano: The most efficient in the Gemini lineup, specifically designed for on-device applications. Gemini Nano comes in two versions, Nano-1 and Nano-2, with 1.8B and 3.25B parameters respectively, targeting low and high memory devices. By employing advanced model distillation techniques and 4-bit quantization for deployment, the Nano models provide outstanding performance for on-device applications. The only model in the same category reportedly surpassing its performance is Phi-2 [source].
Performance Benchmarks
The Gemini Ultra model represents a significant leap in AI capabilities, as evidenced by its exceptional performance across a wide range of benchmarks. Key highlights include:
- State-of-the-Art Results: Gemini Ultra sets a new benchmark in AI performance, excelling in 30 out of 32 evaluated areas. This encompasses various domains, including text and reasoning, image and video understanding, speech recognition and translation. Notably, it achieves a groundbreaking feat by being the first AI model to surpass human-expert performance on the MMLU exam benchmark, underlining its remarkable capability in complex multimodal tasks.
- Mathematics and Coding Mastery: In specialized benchmarks like GSM8K and HumanEval, Gemini Ultra consistently outperforms existing models. This highlights its superior analytical and problem-solving skills, making it an invaluable tool in fields that demand high-level mathematical and coding proficiency.
However, readers are advised to consider these highlighted results in light of the controversies discussed further in this article, which call for a careful examination of Google’s claims and remind us of the need for independent verification of such benchmarks.
Controversy Surrounding the Model
The unveiling of Google's Gemini model has not been without its share of controversy, highlighting the complexities and challenges in presenting and evaluating cutting-edge AI technologies. Two major points of contention have emerged, drawing significant attention and critique from the AI community.
- Misleading Demonstration Video: A key issue that arose was related to a demonstration video presented by Google, which was later admitted to have been altered. Google conceded that the video was edited to showcase the Gemini model in a more favorable light, leading to accusations of misleading the public about the model's actual capabilities.
- Selective Benchmark Reporting: Another significant controversy involves the selective reporting of benchmark results. Multiple sources highlighted that while Gemini was shown to excel in certain benchmarks, there were notable omissions in Google's presentation, particularly benchmarks where GPT-4 had an edge over Gemini.
Comparison with GPT-4
The benchmarks released by Google suggest that Gemini may outperform GPT-4 in certain reasoning and math tasks, yet these results should be met with a healthy dose of skepticism. Given the recent controversies, including the use of an edited video and selective benchmark reporting, Google's credibility in presenting their model's capabilities has been called into question. Until these results can be independently validated, it's prudent to reserve judgment and consider the full context of Gemini's performance relative to GPT-4, acknowledging the broader discussion about accurate and unbiased AI model evaluation.
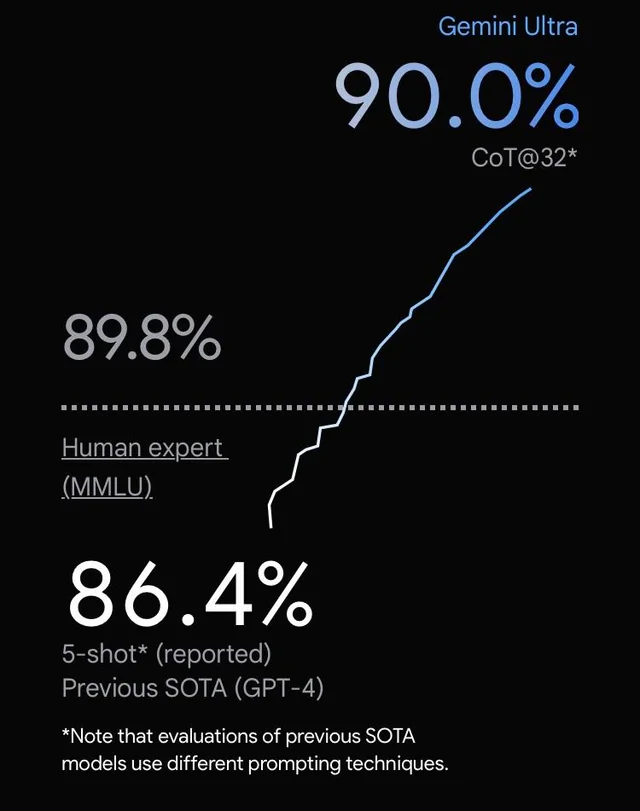
Conclusion
The introduction of Google's Gemini model to the competitive landscape of Large Language Models, with its advanced multimodal capabilities, is a noteworthy event. Its impressive performance could be a game-changer if further evaluations uphold Google's claims. However, the model's true standing, particularly in comparison to GPT-4, will hinge on unbiased, independent validation in the times ahead.