South Korea Enters the LLM Arena with a bang!
Stefan Wendin had the remarkable chance to meet Hwalsuk Lee, the Chief Technology Officer at Upstage, for a stimulating and informative lunch in Seoul. Our gathering occurred in a unique, traditional setting - a Korean BBQ place in the Banpo underground shopping mall's basement. This hidden culinary treasure offered an exceptional experience and set a conducive atmosphere for our comprehensive discussion about Large Language Models (LLMs) and AI intricacies.
During our conversation, we focused particularly on the SOLAR 10.7B model, which captivated me due to its innovative yet straightforward DuS approach and its efficient performance despite limited memory requirements. We delved into how benchmarks are crucial for initial assessments but emphasized the significance of a model's resource efficiency, architectural complexity, training, and fine-tuning. And despite the emergence of numerous new (hybrid) models, the original 10.7B stands out as a leader in the field. This invaluable exchange of insights was made possible thanks to Petr Kazar, whose introduction was instrumental in facilitating this meeting.
The recent unveiling of SOLAR 10.7B by Upstage marks a significant milestone in the field of Large Language Models (LLMs). Distinguished by its unique Depth Up-Scaling (DUS) approach (explained below), SOLAR 10.7B integrates the robust architecture of Llama 2 with the advanced capabilities of Mistral 7B. This article aims to provide an insightful overview of SOLAR 10.7B by examining its architectural innovation, training methodology, and performance metrics, thereby shedding light on its potential impact and role in advancing natural language processing and AI.
Architectural Innovation in SOLAR 10.7B
SOLAR 10.7B's distinctiveness lies in its implementation of Depth Up-Scaling (DUS), a method that expands the model's processing capabilities by adding more layers to its existing neural network. Beginning with a 32-layer Llama 2 architecture, SOLAR 10.7B integrates the pretrained weights from Mistral 7B, creating a unique combination that leverages the strengths of both models.
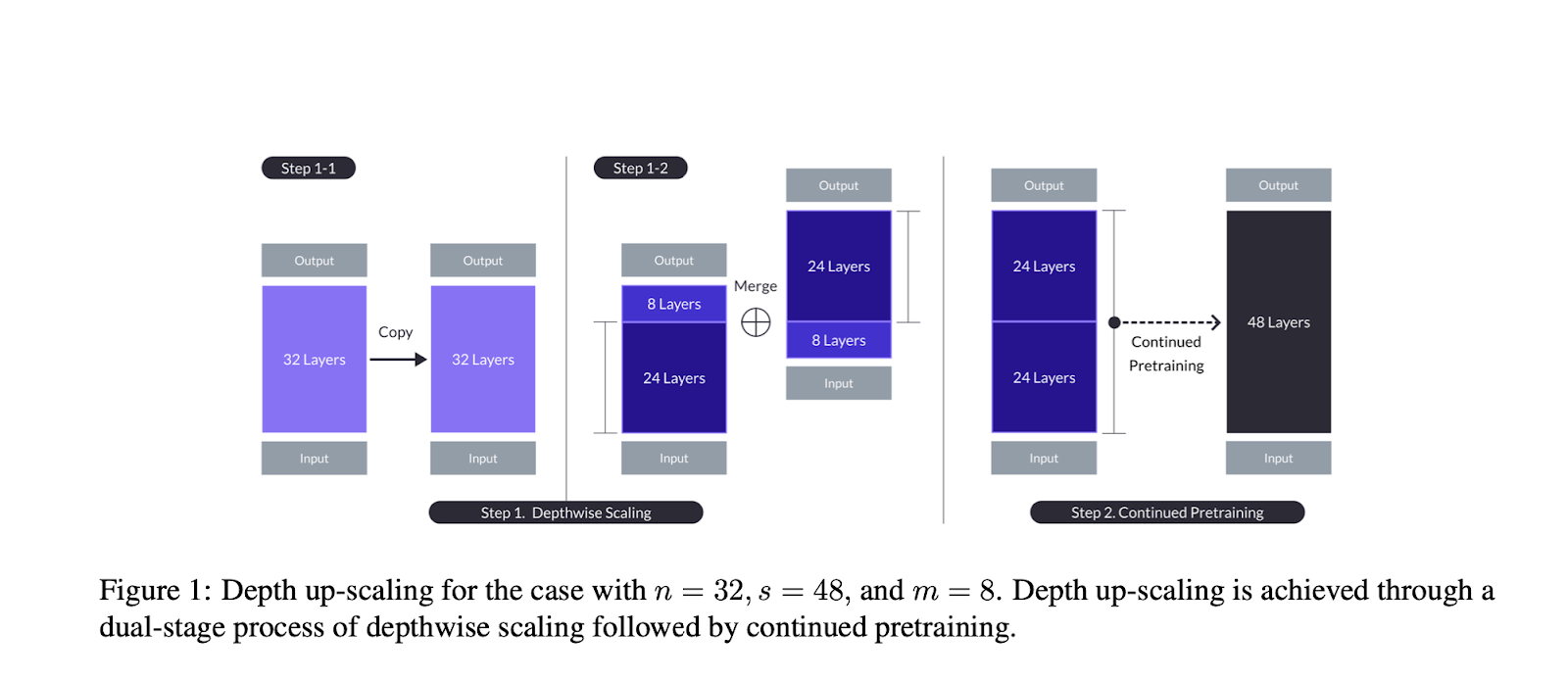
The DUS approach is a strategic decision to enhance the model's depth rather than its width, focusing on adding processing layers. This method increases the model's language processing abilities while maintaining the size relatively small. It's a subtle yet effective way of enhancing model performance, which may seem straightforward but requires precise execution to maintain balance and efficiency.
Training Methodology of SOLAR 10.7B
SOLAR 10.7B's training approach is a meticulous process that involves two crucial stages: instruction tuning and alignment tuning. These stages are designed to not only enhance the model's language processing capabilities but also to align its outputs with ethical and societal standards.
- Instruction Tuning: This first stage is pivotal in developing the model's core ability to understand and follow complex instructions. It involves training the model with diverse datasets specifically curated to improve responsiveness to a wide range of commands. This phase lays the foundation for SOLAR 10.7B’s interactive and responsive capabilities.
- Alignment Tuning: The second stage is where SOLAR 10.7B is fine-tuned to produce outputs that are ethically sound and contextually appropriate. This phase employs datasets that contain dialogues and scenarios aimed at aligning the model's responses with ethical considerations and human values. It ensures that the model's interactions are responsible and socially aware.
Performance
The performance of SOLAR 10.7B, especially when benchmarked against contemporary models, is noteworthy. It surpasses models of similar sizes, like Qwen 14B and Mistral 7B, demonstrating the effectiveness of its Depth Up-Scaling (DUS) method. Particularly, SOLAR 10.7B-Instruct, despite its smaller size, achieves the highest Model H6 score, outperforming even the larger Mixtral 8x7B-Instruct-v0.1 and Qwen 72B. The H6 score is a metric evaluating a model's proficiency in single-turn conversations, assessing its ability to understand and respond accurately in a single interaction. These results solidify SOLAR 10.7B's position at the forefront of current open-source LLMs, showcasing its superior design and efficiency.
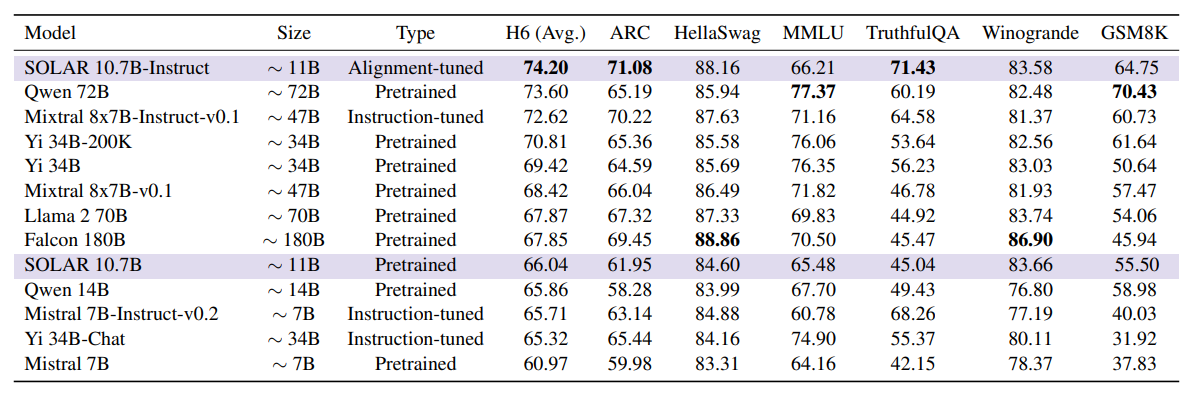
Conclusion
SOLAR 10.7B's introduction showcases a transformative step in LLMs, blending Llama 2's architecture with Mistral 7B's weights for unparalleled performance. Notably, its success in single-turn conversations, as reflected by its impressive Model H6 score, marks a new industry benchmark. This breakthrough underscores South Korea's rising prominence in AI, promising innovative applications of LLMs across diverse fields.