Using the Language Powerhouse for Effective Content Generation
Large Language Models are the text generation powerhouse of current times. The powerful natural language understanding of these models coupled with the continuous advancements and integrations with major tech-stacks make this an exciting time to think about adopting them for driving effective processes and business value.
Incorporating LLMs into content creation workflows can lead to a huge efficiency boost for businesses and professionals, which leads to more impact generation. These models not only provide automated generation, but when used effectively, they can also give more control over the output quality, writing style, language tonality which can lead to interesting information variety and personalization.
Our team at Predli is really excited about the ongoing developments and we conduct extensive research and scope out the latest releases with the aim of creating State-of-the-Art products, which can simplify the lives of our end-users. One such product we worked on was around Generation from Seed-Data, which we will cover in this blogpost.
We designed our experiment to test the LLMs’ content generation capabilities with a heavy focus on controlled and guided generation. An ideal case scenario would be where subject matter experts/creators provide a short seed data leading to content generation. It would enable fast paced iterations and experimentations to hit the perfect combination of various content parameters. For instance, upon giving the context of a press conference in the form of short notes taken by an attendee, our system should generate structured composition for a news article. Another instance would be formulating sections for an annual sustainability report /financial report by providing a crisp layout of the firm’s vision. Not only that, since we are generating the final results from user-defined seed data, the results don’t count as AI Generated.
Our Approach
We divided our task into two steps: finding the appropriate seed data for desired content matter and effective generation from the seed. For our exploration, we took various excerpts of existing textual content for extracting seed data, generated content using the seed and compared/evaluated the results. The process involved a lot of iterations, for coming up with a structure to capture the required context in a simple and crisp seed as well as provide experimental flexibility to the user. Our targeted seed structure was something that the user can fill in a few minutes and then experiment with a variety of content generation depending on the use case.
One thing we wanted to ensure while working on this was that the model can be generalized to generate content in any requested format, based on all kinds of seed data. We ultimately decided to use LLMs (ChatGPT 3.5 turbo, in our case) for making a proper seed structure. The idea was to capture the appropriate structure by providing existing text in the model’s context window and guiding the model efficiently using methods like Chain of Thought (COT) prompting and Few-Shot Inference generation. We used the few-shot approach for text generation based on seed data and found that GPT was powerful enough to pick on the pattern for specific use cases and generate similar content.
Another interesting challenge posed out to define a good evaluation metric for quantifying the generation quality with respect to the provided excerpt. We tested both syntactic (BLEU, ROUGE) and semantic metrics (BERT Score) along with manual evaluation. Additionally, it was fun playing with the idea of G-Eval for evaluating natural language generation. G-Eval involves using powerful models like GPT4 for evaluating generated output quality and has more correlation with human evaluations as compared with other methods.
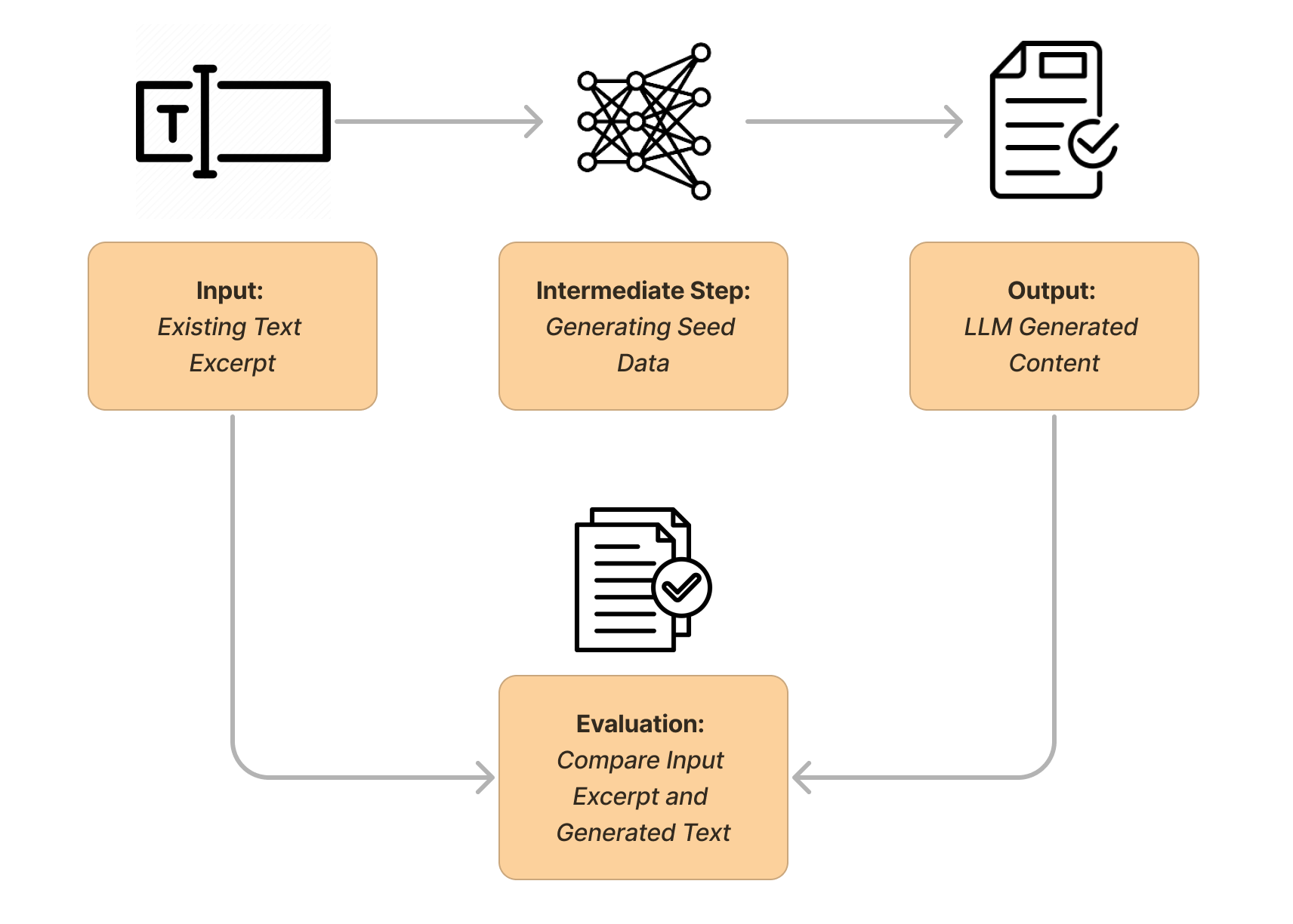
We found that GPT3.5 was able to infer the language and generate content using seed which was similar to the provided text excerpts.
Observations/Learnings:
We had a great time exploring the capabilities of state of art language models and the associated content generation use cases they can be applied to. Using these powerhouses effectively is as much an art as it is science. Overall observations and learnings from our explorations are broadly discussed as following:
- Recent LLMs possess a great command over various content generation capabilities. They shine at creative tasks, give impressive performance by appropriately incorporating provided context and show versatility in generating a variety of content. They present an exciting option to be included in the process of generating content, providing ways towards content personalization and fast iterations.
- LLMs basically work on the principle of autoregressive generation (i.e., generating next token based on the existing sequence). Hence, they work towards generating the sequence of words which makes more statistical sense and that can sometimes be different from the intended text (broadly known as hallucination). It becomes imperative to guide the generation using effective methods which in our case majorly happens through the seed data.
- Controlling the length, writing style, tonality and coherence between various generations is also crucial for squeezing maximum productivity and practical use from this technology. We have included the associated variables and modified the model behavior using few-shot learning to deal with this challenge.
- There can sometimes be information seep in from few-shot examples which might be incorrectly used while content generation for a different firm/section/context. Problems like these can be identified using proper testing mechanisms and rectified by prompt optimization.
- In our opinion, LLMs should be treated like skilled assistants/co-pilots rather than content generators. They definitely have the potential of changing the process of content creation with effective guidance and refinement.
We at Predli understand the tremendous potential of large language models to transform businesses. As leading experts in this emerging field, we stay on the cutting edge of new developments so that we can harness the latest innovations to solve our clients' most pressing challenges. We are actively exploring state-of-the-art developments to uncover new ways to extract insights, automate processes, and create unique customer experiences.
This is an incredibly exciting time as artificial intelligence is fundamentally changing how we interact with technology, and we are passionate about being a part of shaping that future!